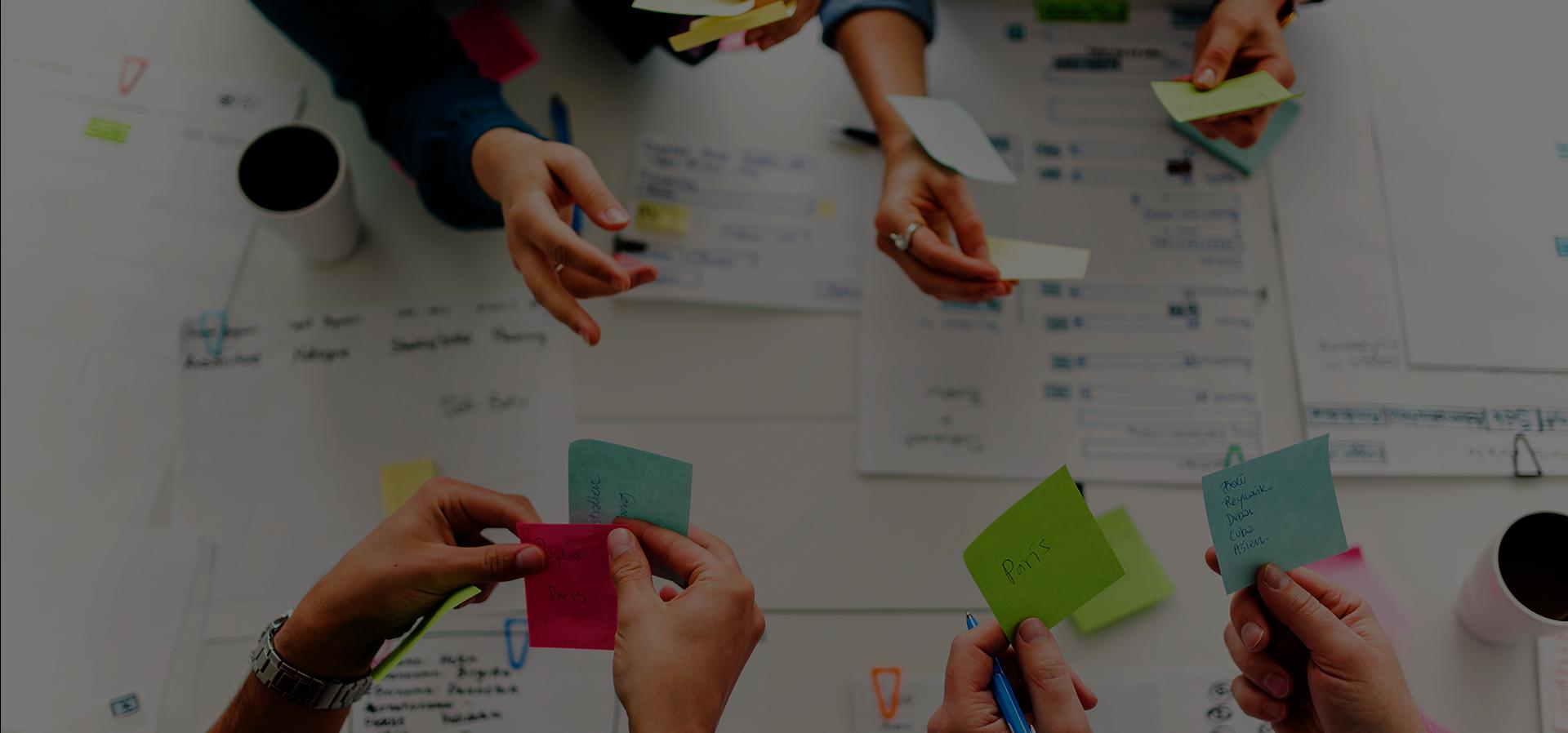
Resources
Publications
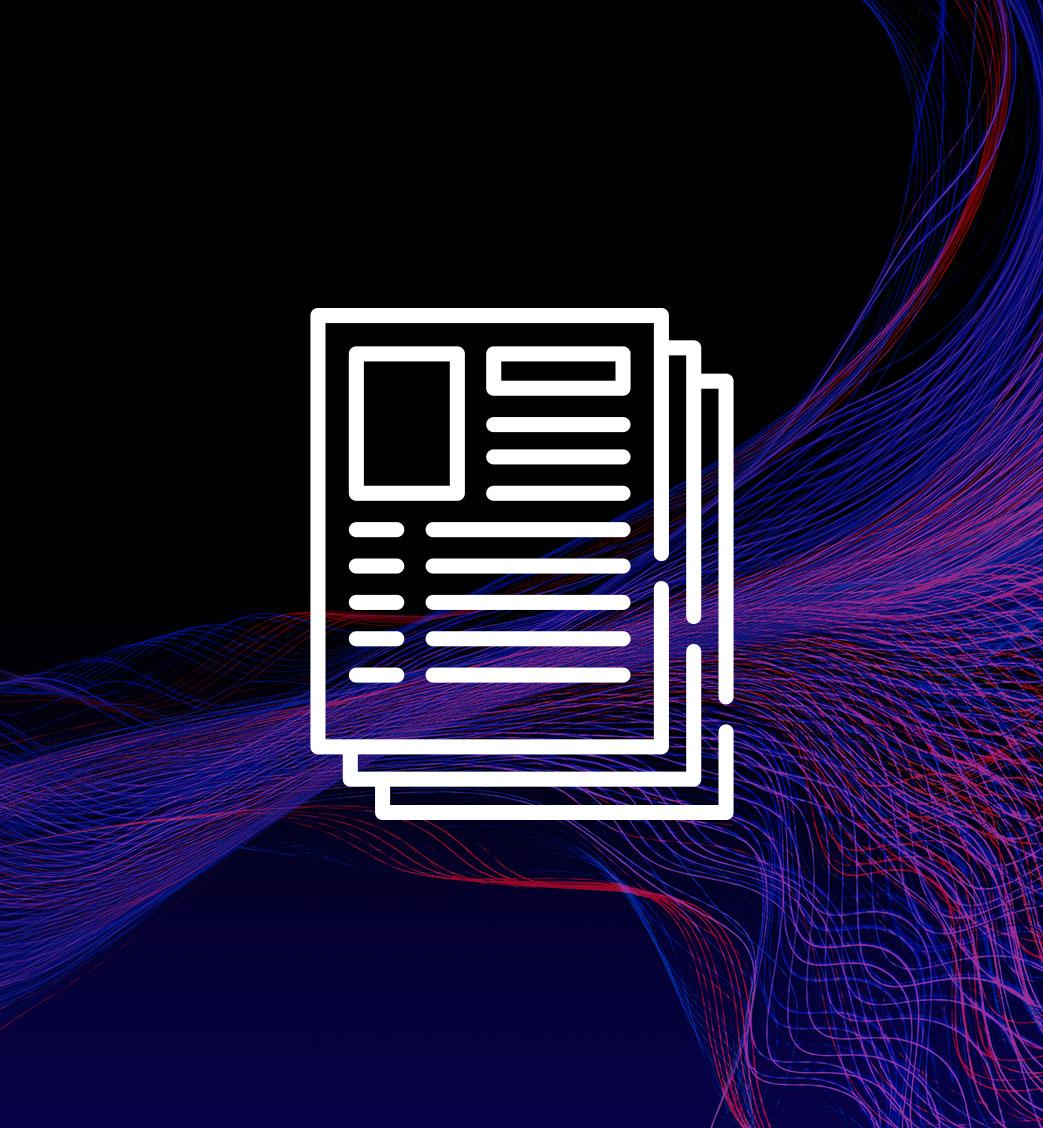
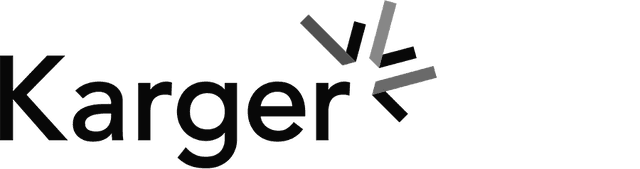
Our Position on Digital Therapeutics
The Problem:
Depending on who you ask, anything from an online dosing calculator to an AI-enabled chatbot qualifies as a digital therapeutic. Because of this ambiguity, health care providers have been slow to adopt what many consider to lack in the rigor of traditional therapeutics, and investors remain hesitant to support what may or may not be a legitimate application of computation to therapeutics.
The Solution:
In a peer-reviewed publication, we posit that a true digital therapeutic should effectively incorporate an artificial intelligence or machine learning-based analysis of digital biomarkers via a closed-loop system to directly improve patient outcomes. Just being a digitized version of an existing therapeutic isn’t enough.
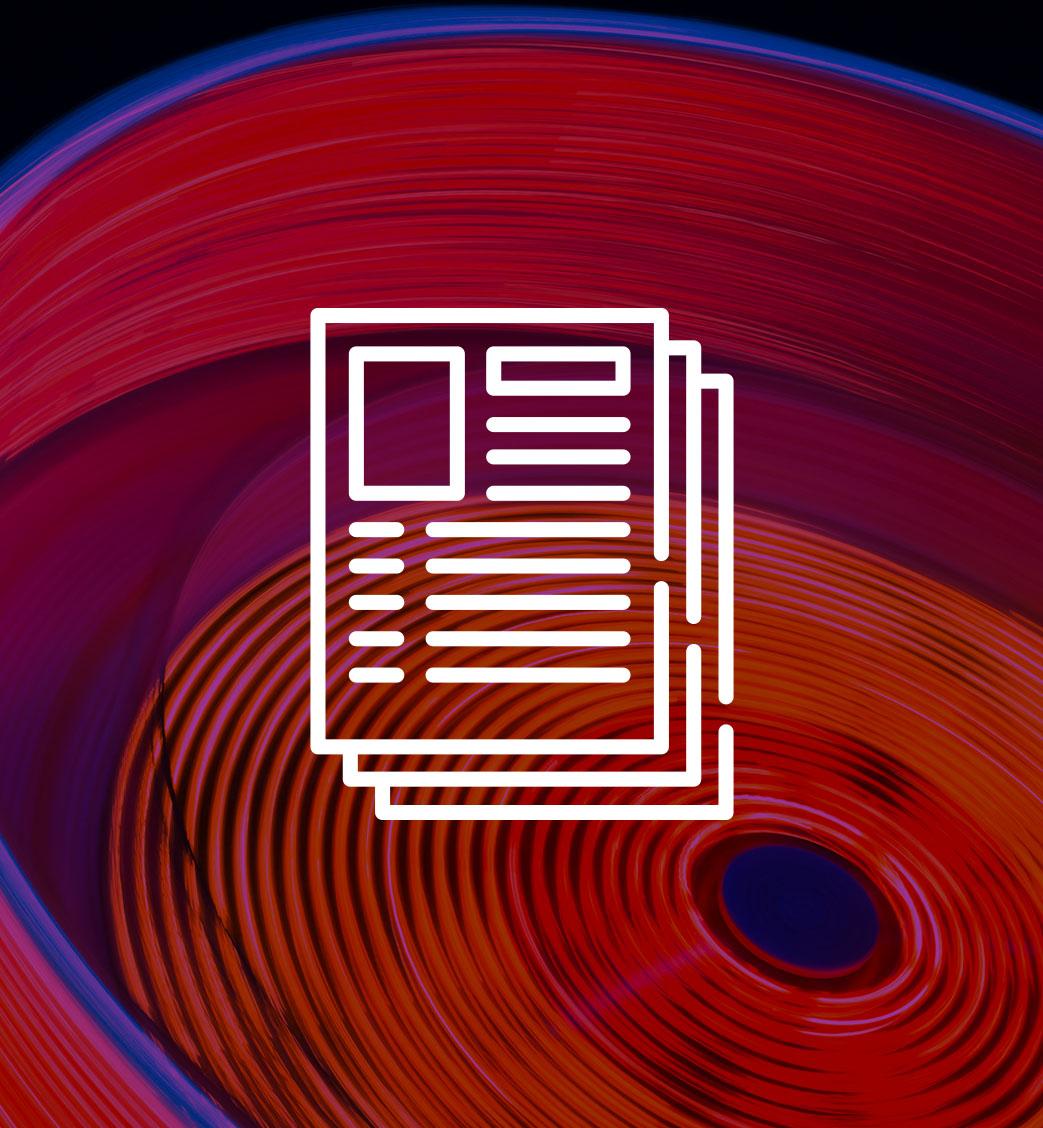
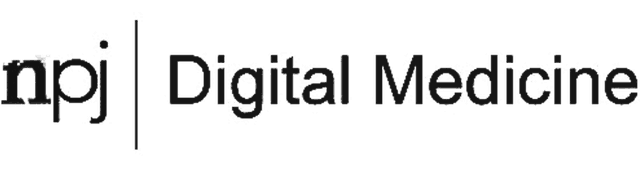
Voice Assistants In Health
The Problem:
From dedicated home assistants to integrated smartphone and automobile-based voice analysis, people are increasingly relying on voice assistants to both send and receive information. But the vocabulary used to health is more complex than typical use cases, and the ability of voice assistants to understand the precise meaning of questions in healthcare can have dire consequences when not executed perfectly.
The Solution:
Through exhaustive testing a list of the 50 most common branded and generic medication names, and controlling for regional accents, we found that Google’s voice platform drastically outperformed both Apple’s Siri and Amazon’s Alexa in recognition of medication names. These results were peer-reviewed and published in Nature Digital Medicine.
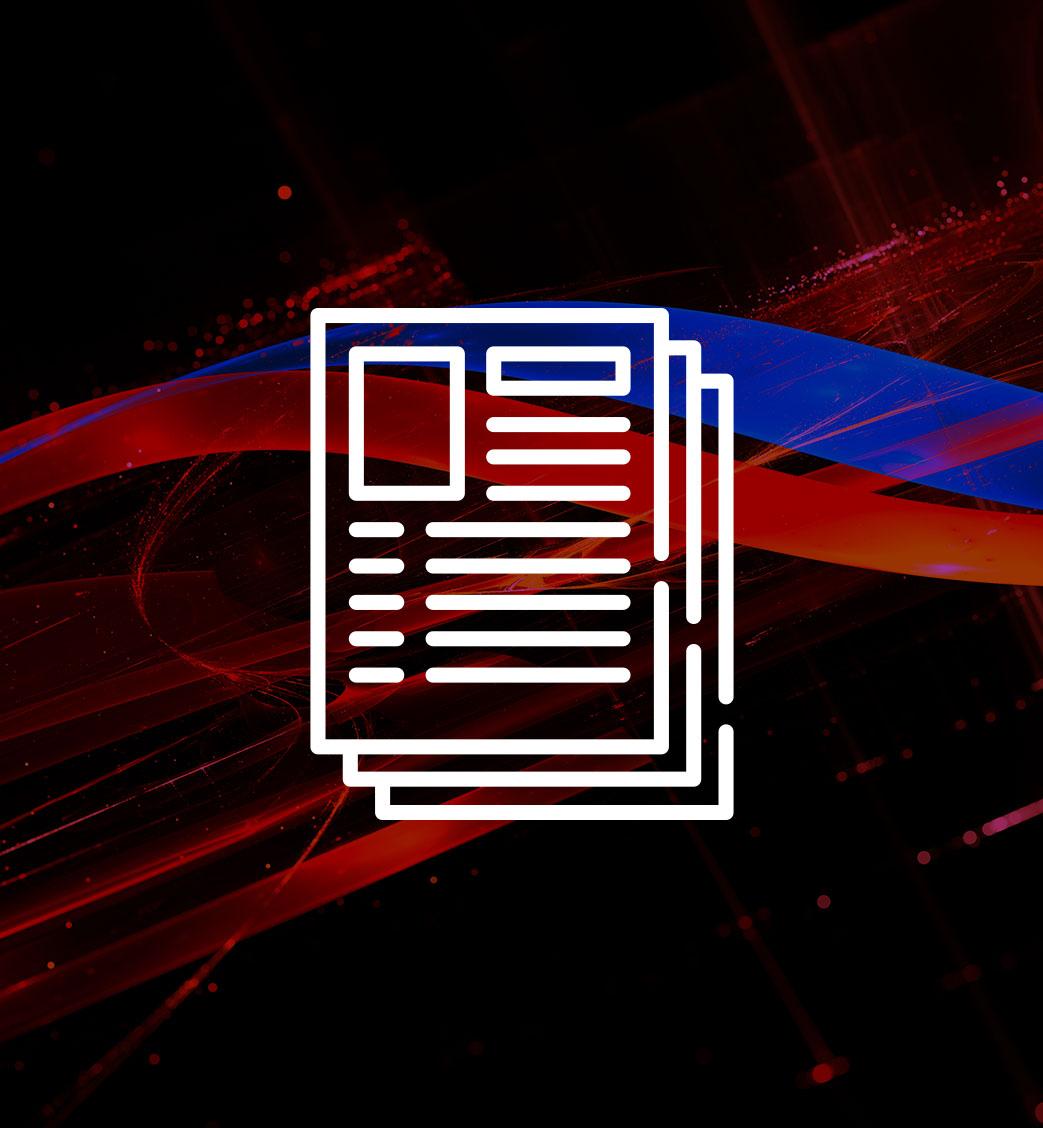
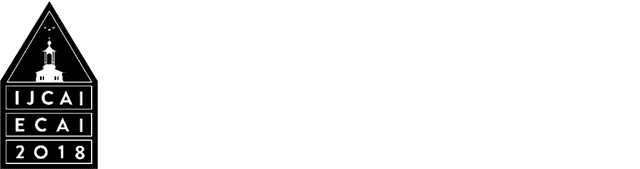
Applying Machine Learning to Physiological Predictions
The Problem:
The "artificial pancreas", a closed-loop system where a continuous glucose monitor automatically administers insulin to people with diabetes, has been a goal in diabetes management for decades. But the ability to predict someone’s blood glucose, even 30 minutes into the future, has eluded researchers. This fundamental lack of understanding has made the goal nearly impossible to realize.
The Solution:
Trying a number of different learning approaches, our data science team used its clinical expertise and quickly realized that much of the input data signals could be effectively discarded, along with outlier data that didn’t make clinical sense. We went on to develop the winning predictive model in the Blood Glucose Challenge, effectively looking 30 minutes into the future of a patient’s blood glucose levels solely based on their previous levels with over 95% accuracy.
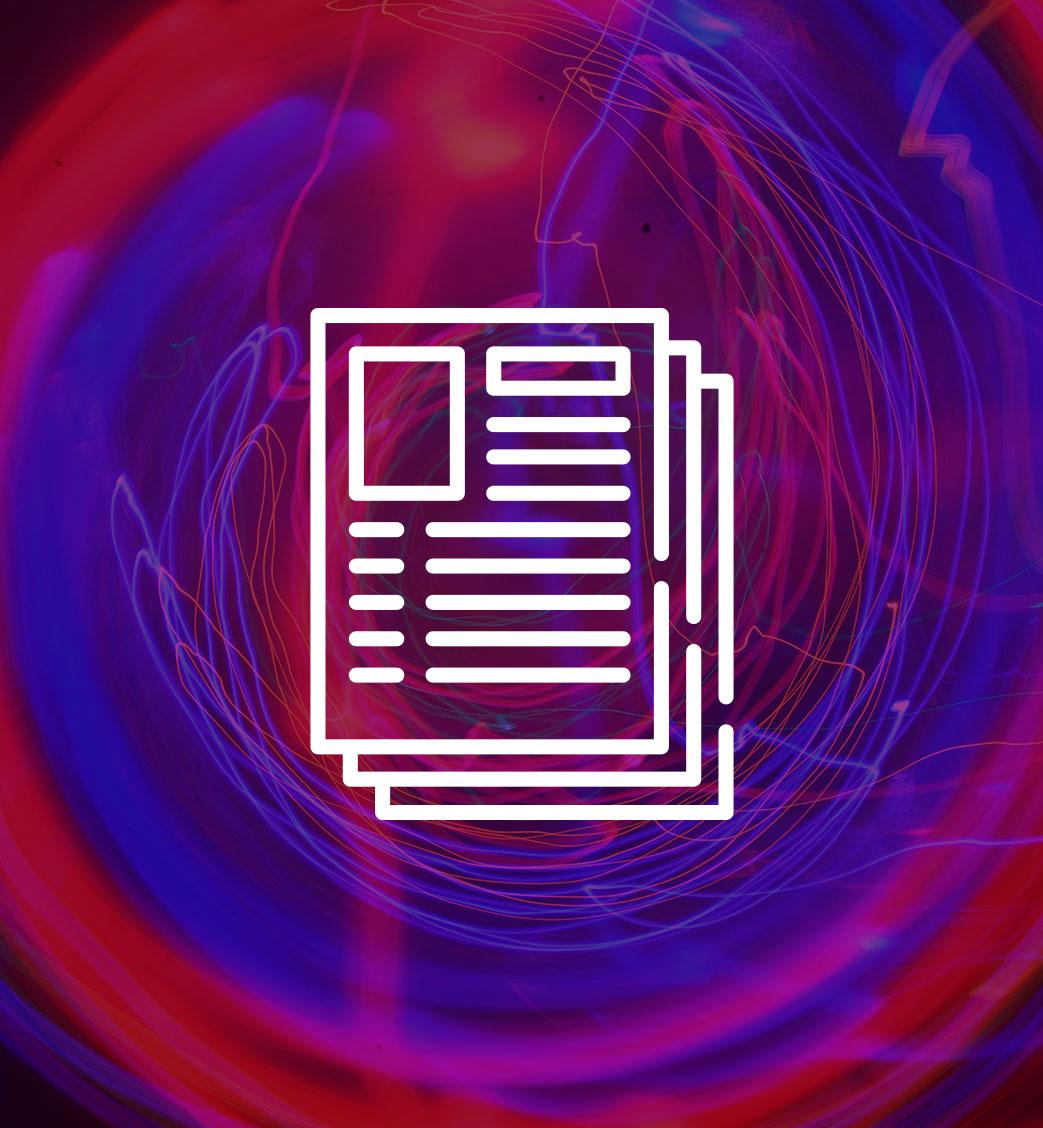
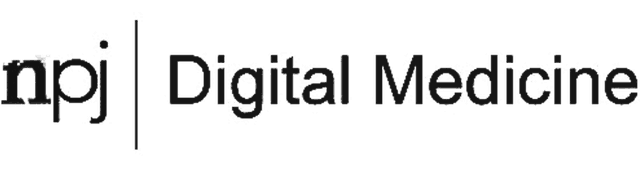
A New Way to Model Physiological Glucose Levels
The Problem:
Mathematical models of physiological processes often suffer from being overly complex as they attempt to model all factors that feed into a physical output. This complexity reduces their utility in a clinical setting, and clinicians are often reliant on cruder measurements like the oral glucose tolerance test to diagnose complex conditions.
The Solution:
Rather than attempting to model the underlying processes that lead to glucose regulation in healthy individuals, we treated the body as a “black box” and modeled the only parameter that matters: blood glucose levels. We did this by recording continuous glucose measurements from healthy volunteers and applied the engineering principles of a proportional integral controller system (the same type used for torpedo guidance systems and thermostats) to give us a general glucose homeostasis function that reliably models the output of the physiological system, regardless of daily activity or physical characteristics.
Our model also allows us to potentially tease apart new types of glucose dysregulation that had previously been lumped together simply as “diabetes,” and offers a potentially simplified and more accurate means of diagnosing people with prediabetes.